The time series analysis is a central element of statistics, especially in economic contexts. In order to develop a solid understanding of error analysis and quality estimation in Excel, you will be guided through a practical application case in this guide. This will be done using the example of a case study of an automotive supplier. You will learn how to compare forecasts with actual values and quantify the quality of your forecasts through error analysis.
Main Insights
- You will learn how to compare forecasts and actual values in Excel.
- You will find out which error metrics are used to evaluate the forecast quality.
- By the end, you will be able to calculate the coefficient of variation and the Root Mean Square Error (RMSE).
Step-by-Step Guide
Start by entering the 2019 values as forecasts and the 2020 values as actual values in Excel. Make sure to accurately transfer the numbers to establish a solid basis for your calculations.
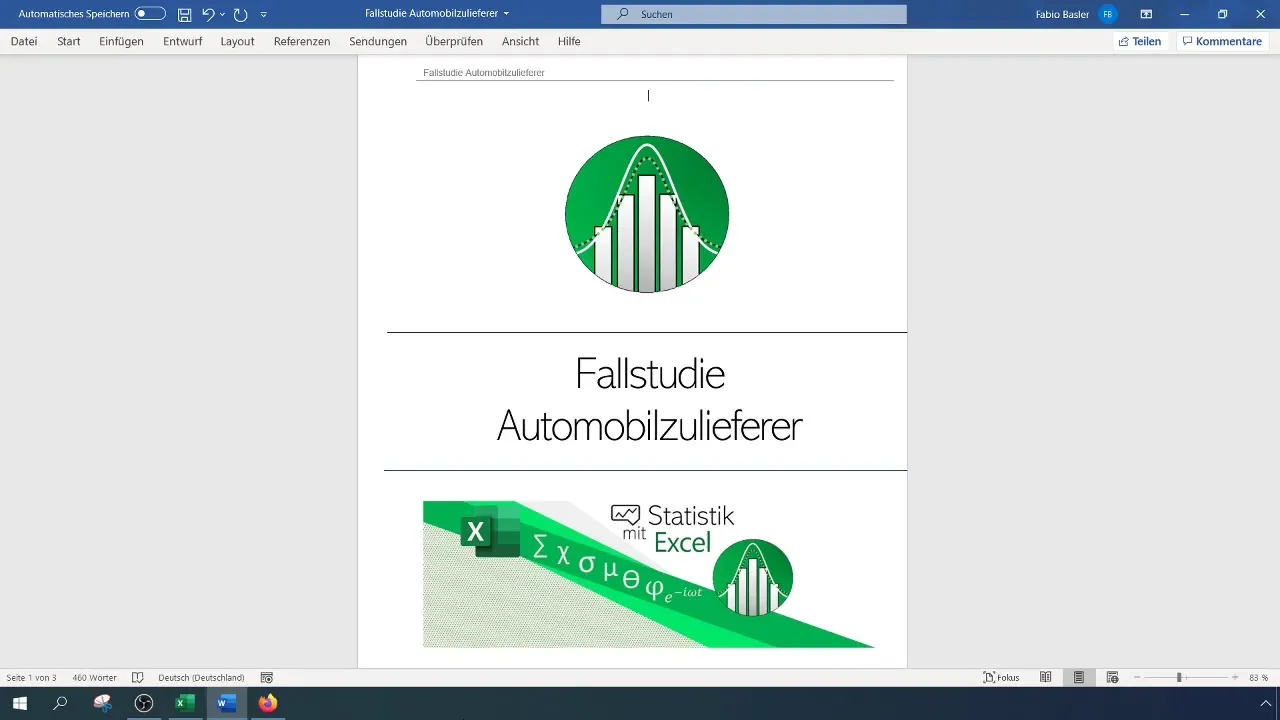
To conduct the analysis, you will need the raw data for both years. You should verify the 2020 numbers and then integrate the forecast values for 2019. These values will serve as the basis for your calculations.
Now, copy the raw data of the 2020 numbers into a working area and paste them in full. To keep the calculations well-structured, it is advisable to create separate columns for forecasts and actual values.
In the next step, you need to subtract the forecasts from the actual values to calculate the errors. To do this, use the formula "Error = Actual Value - Forecast". Apply this calculation across all your data points to quantify all errors.
After calculating the errors, the next step is to square these errors. This means multiplying each error by itself, which will provide you with the squared errors.
Now, calculate the mean of the squared errors. To do this, use the "Average" function in Excel and divide the sum of squared errors by the number of observations. This will give you the average squared error.
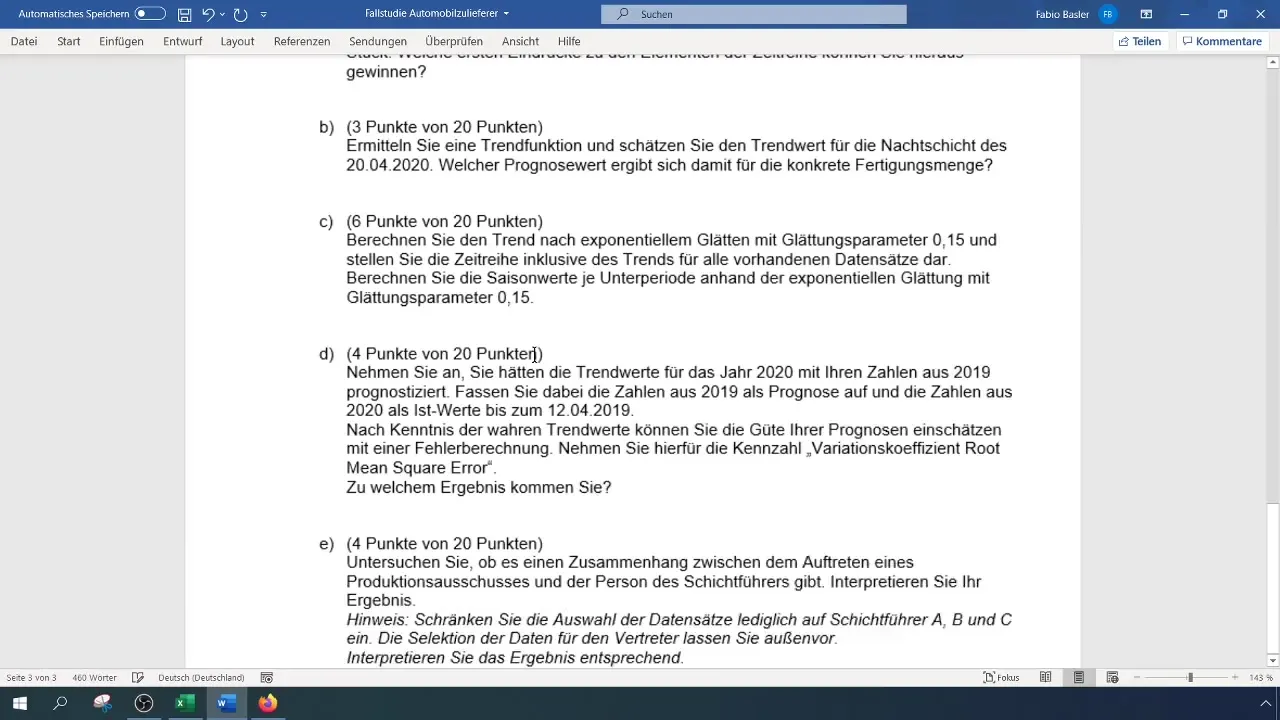
Once the mean of the squared errors has been determined, take the square root of this mean. This leads to the Root Mean Square Error (RMSE). This value is crucial for evaluating the quality of your forecasts.
Now you also want to calculate the average of the actual values. Again, use the "Average" function and select the relevant actual values. This average is important for the subsequent interpretation of the coefficient of variation.
In the next step, calculate the coefficient of variation (CV). The CV is calculated by dividing the RMSE by the average of the actual values. This gives you a percentage representation of the errors compared to the actual values, assessing the quality of your forecasts.
The interpretation of the coefficient of variation is essential. A CV of 0.08 indicates a low relative variation and thus high forecast accuracy. You can enter this number and your insights into a reference table to make the results more understandable.
In summary, you have analyzed forecasts and actual values in Excel through several steps. Calculating errors, squaring them, computing averages, and ultimately determining the coefficient of variation are fundamental procedures for evaluating the quality of time series analyses.
Summary
In this guide, you have explored how to analyze time series in Excel by comparing forecasts with actual values. You have learned how to calculate errors, square them, and quantify the quality of your forecasts. By determining the coefficient of variation, you now have the ability to better assess future forecasts.
Frequently Asked Questions
How can I present actual values and forecasts in Excel?By creating separate columns for actual values and forecasts, and entering the corresponding values into these columns.
How do I calculate the RMSE?The RMSE is calculated by taking the square root of the mean of the squared errors.
What does a high coefficient of variation indicate?A high coefficient of variation indicates a high relative variation, which implies lower forecast accuracy.
Why is it important to square errors?Squaring errors ensures that positive and negative deviations do not cancel each other out when calculating the mean.