Statistical analyses are an essential tool in portfolio management. One particularly powerful technique is regression analysis, especially when it comes to determining the influence of multiple independent variables on a dependent variable. Do you want to learn how to build and analyze a linear functional relationship through multiple regression in Excel? This guide offers you a clear overview and a detailed step-by-step guide.
Main Insights
- Regression analysis helps quantify the impact of independent variables on a dependent variable.
- It is important to evaluate the goodness and statistical significance of the regression model.
- By assessing the coefficients, it can be determined which independent variable has a stronger influence on the dependent variable.
Step-by-Step Guide to Regression Analysis in Excel
Preparing the Data
Before you can start with the regression analysis, you need to prepare your data. The dependent variable (y) in this case is the sales of notebooks, and the independent variables (x1 and x2) are the number of employees and the number of competitive actions. First, you should enter all the necessary data into Excel and structure them clearly.
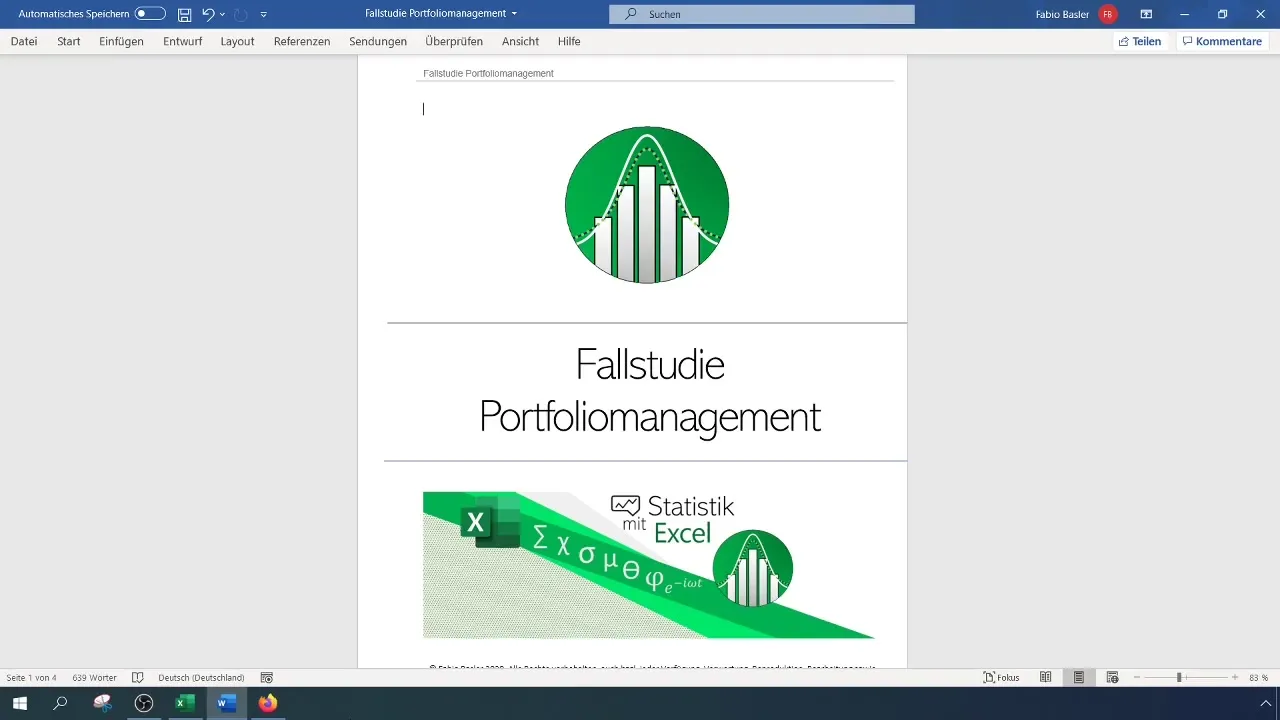
Check for Correlation
To get initial insights into possible relationships between the variables, you can create a correlation matrix. This matrix helps you see how strongly the different variables are connected. You can do this by using the "Data Analysis" function in Excel and then selecting the correlation matrix, including all relevant data ranges.
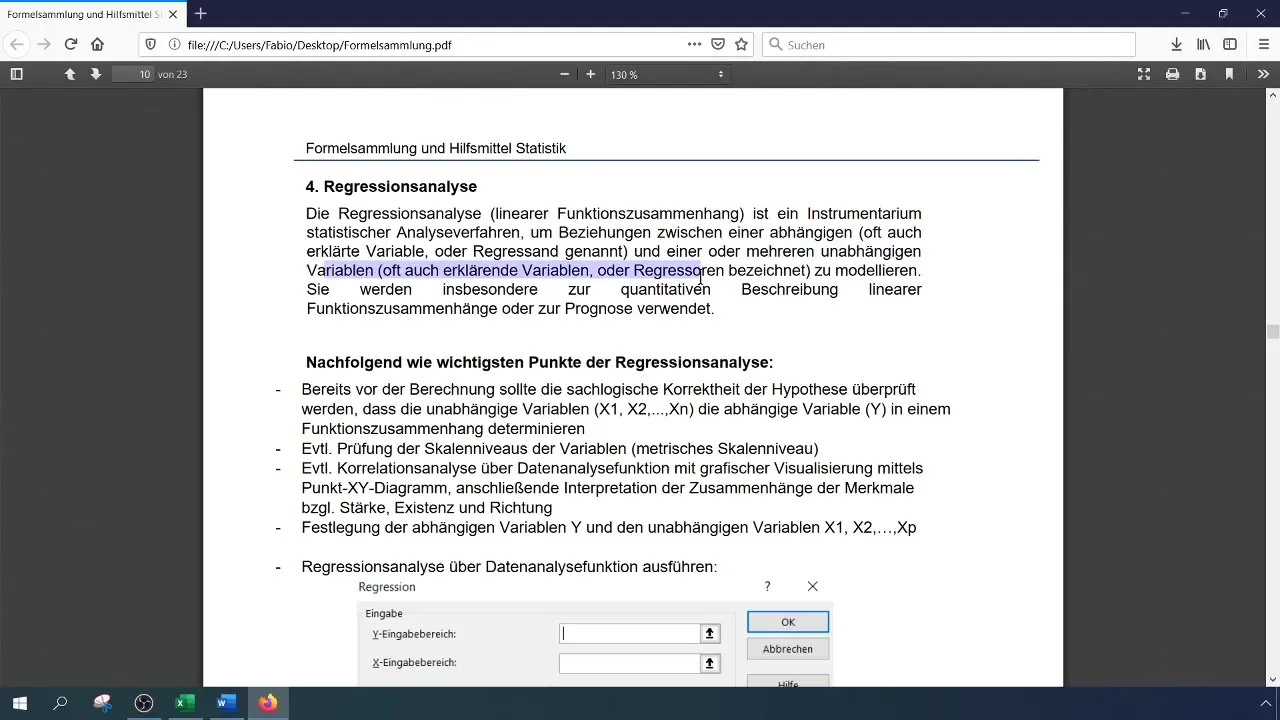
Performing the Regression Analysis
Now you are ready to perform the regression analysis. To do this, again select the "Data Analysis" function, then choose "Regression." Here, you specify the input range for the dependent variable (sales of notebooks) and the independent variables (number of employees and competitive actions).
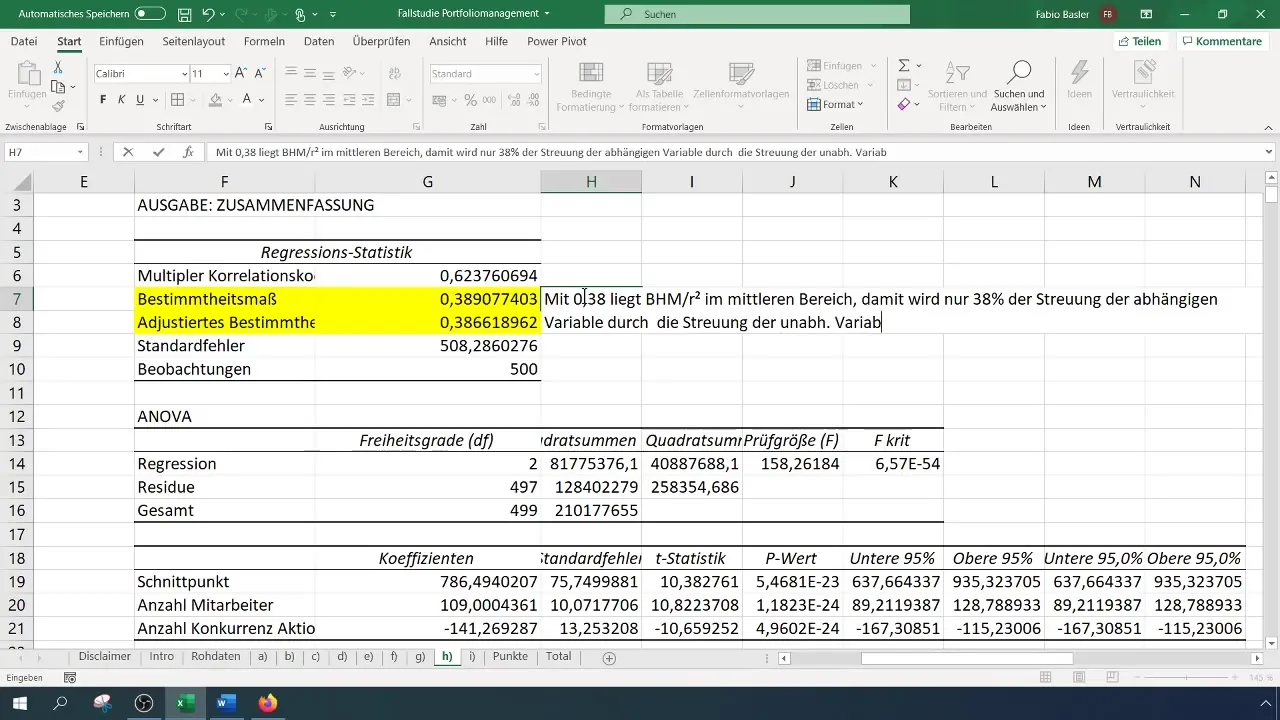
Interpreting the Results
After conducting the regression analysis, you will receive a variety of outputs and statistics. The first important point is the coefficient of determination (R²), which describes the proportion of explanation of the dependent variable by the independent variables. An R² value of 0.38 indicates that 38% of the variability in sales can be explained by the two independent variables.
In the evaluation, pay attention to how stable the model is. Here, you can use the standard error to determine stability. For example, if you get a standard error of 0.51, this indicates high relative variation and thus low stability.
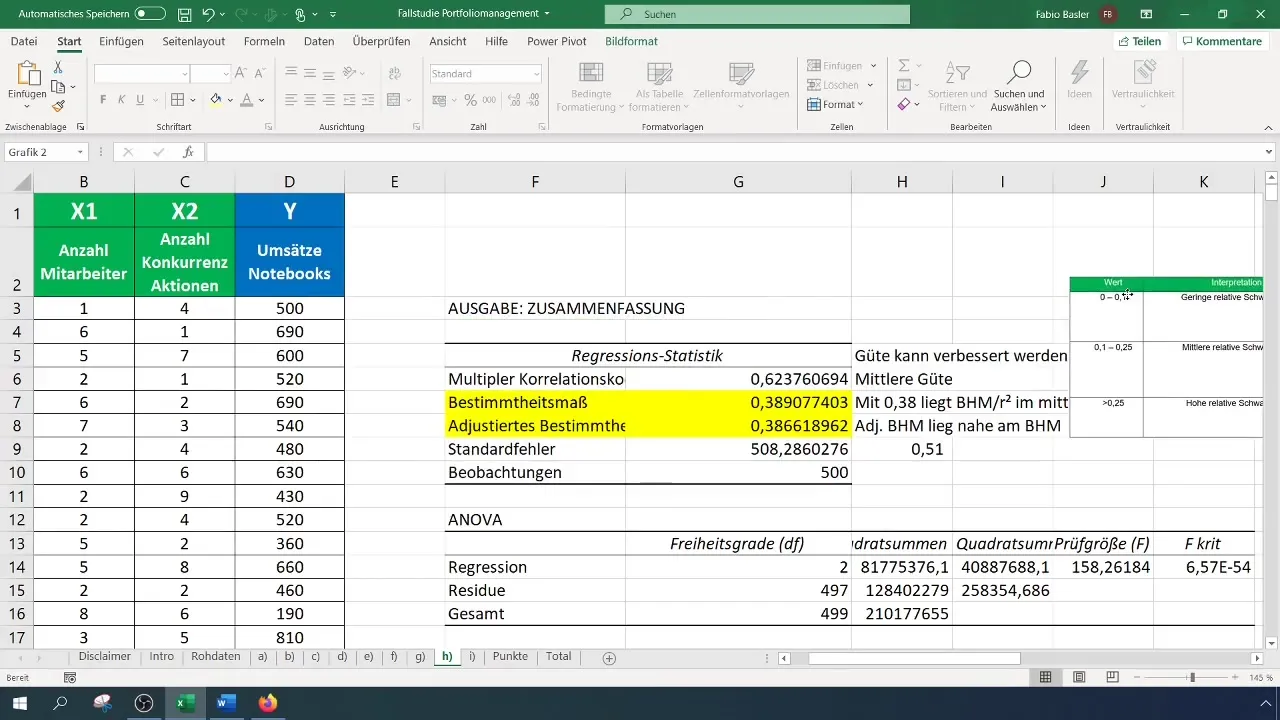
Evaluating Strong Influencing Factors
An essential step is to determine which of the independent variables has a stronger influence on the dependent variable. You can do this by using the coefficients, which indicate the absolute relevance of the variables. In this case, the coefficient of x1 (number of employees) is 109, while the coefficient of x2 (number of competitive actions) is -141. This means that each increase in the number of employees increases sales by 109 euros, while each increase in competitive actions reduces sales by 141 euros.
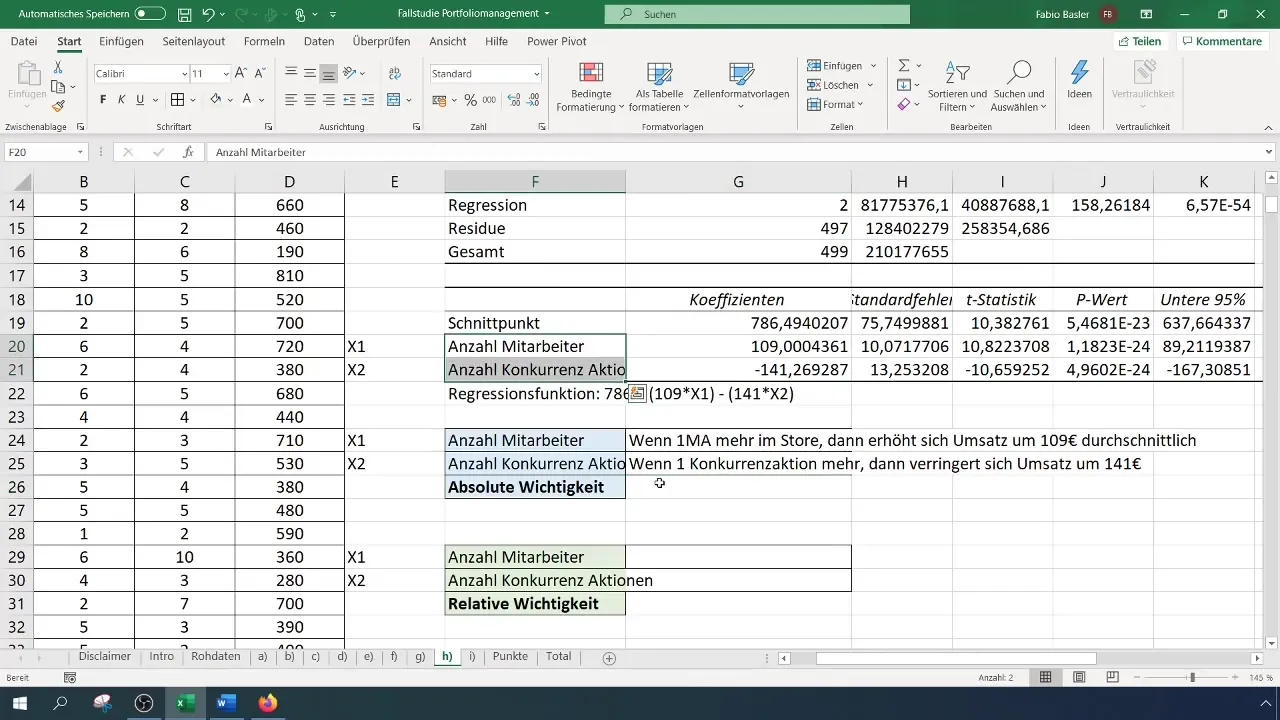
Evaluating the Statistical Significance
Another important aspect of regression analysis is the statistical significance. You can assess this from the p-values and the F-test, both of which should show very small values. This indicates that the independent variables have a significant impact on the dependent variable, justifying the use of the model.
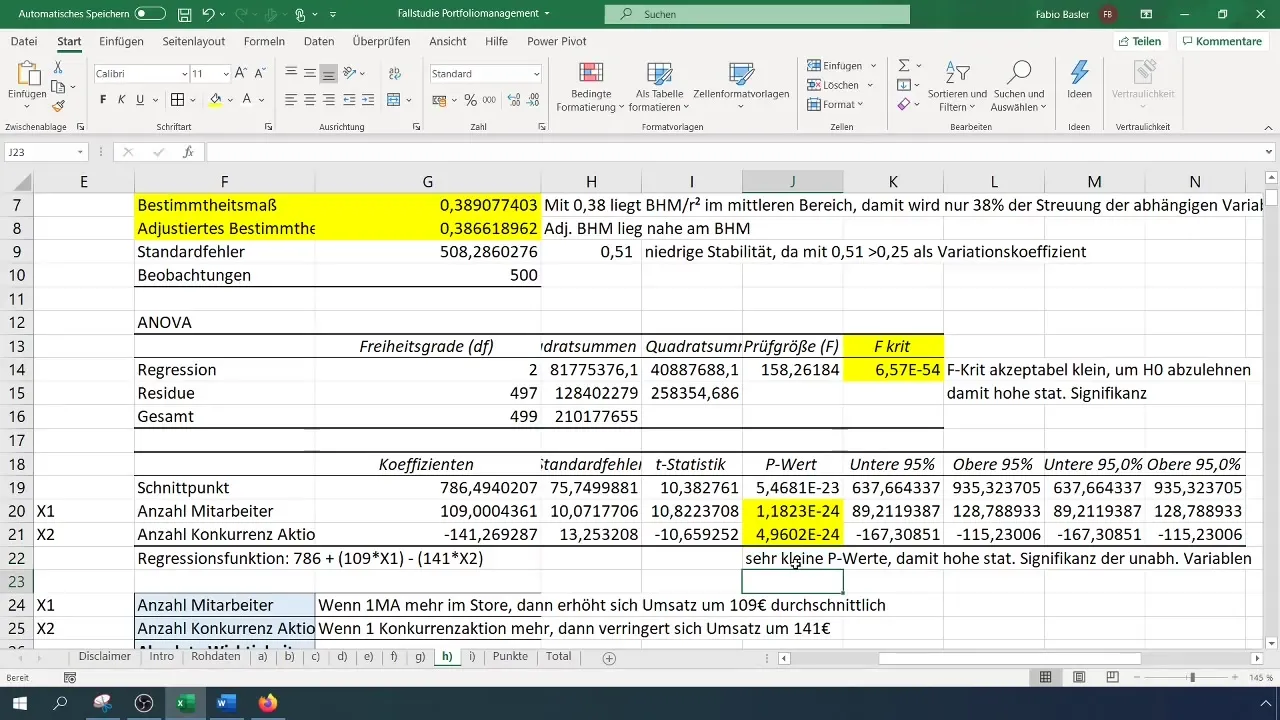
Summary
Regression analysis in Excel allows you to quantitatively understand the relationship between different variables. With this guide, you have learned the steps to perform and interpret a multiple regression. Be sure to critically evaluate the goodness and stability of the model, as well as the individual influencing factors, to make informed decisions in portfolio management.
Frequently Asked Questions
Which data do I need for regression analysis?You need a dependent variable (e.g. revenue) and at least one or more independent variables (e.g. number of employees, competitor actions).
How do I interpret the coefficient of determination R²?A higher R² value means that a larger proportion of the variability in the dependent variable is explained by the independent variables.
How can I test for statistical significance?You can use the p-values and the F-test; low values indicate high significance.
What is the difference between R² and adjusted R²?The adjusted R² takes into account the number of independent variables and provides a more realistic assessment in models with multiple variables.
How can I assess the strength of the influencing factors?This is done by examining the coefficients of the independent variables, which quantify the influence of each variable on the dependent variable.